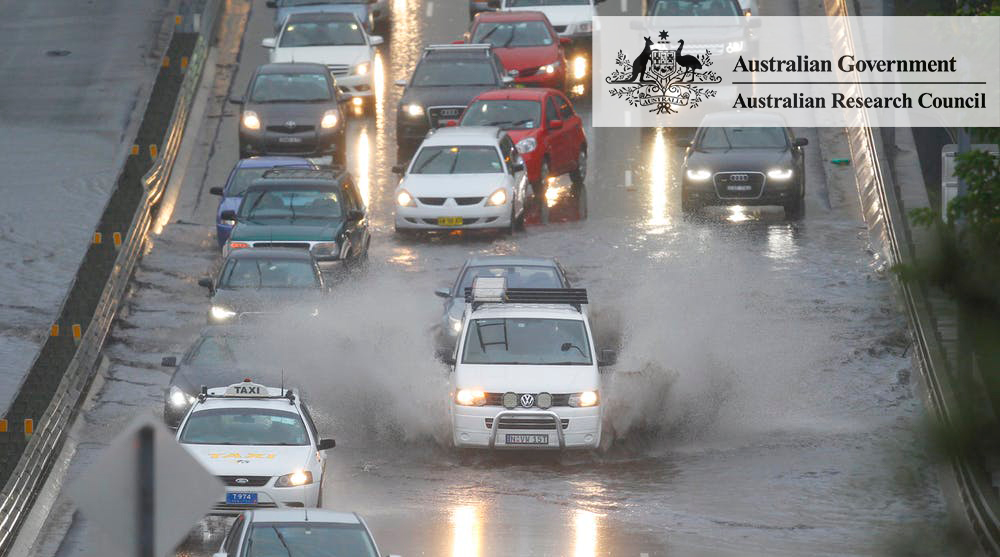
2.56ARC Improving Road Network Operations under Non-recurrent Events (LP170100341)
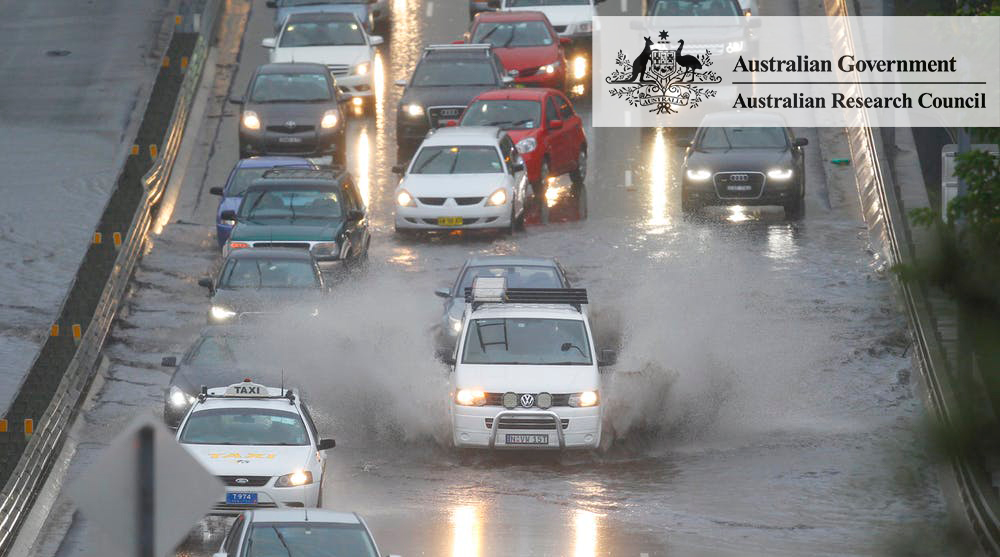
Project Number
2.56ARCRound
Round 5Date
September 2018 - August 2021Research Team
Chair, Project Steering Group
Professor Keith Hampson
BEng(Civil)(Hons) MBA PhD
FIEAust FAIM FAICD
k.hampson@sbenrc.com.au
Chief Investigator
Professor Yong-Hong Wu
PhD
Curtin University
Y.Wu@curtin.edu.au
Project Manager
Assoc. Prof. Benchawan Wiwatanapataphee
PhD
Curtin University
B.Wiwatanapataphee@curtin.edu.au
Documents for Downloading
Presentations
Integrated Control Strategy for Variable Speed Limit (VSL) and Ramp Metering (RM) – Literature Review (March 2019, 615Kb)
Smart Freeway Modelling and Optimization of Traffic Flows (March 2019, 1.5Mb)
Progress Report Presentation (July 2019, 2.5Mb)
Progress Report Presentation (November 2019, 3.5Mb)
Progress Report Presentation (March 2020, 4.2Mb)
On Simulation and Optimization of Freeway Network Operations (June 2020, 4.6Mb)
On Simulation and Optimization of Freeway Network Operations (September 2020, 4.7Mb)
On Simulation and Optimization of Freeway Network Operations (December 2020, 4.2Mb)
On Simulation and Optimization of Freeway Network Operations (February 2021, 4.5Mb)
On Simulation and Optimization of Freeway Network Operations (May 2021)
On Simulation and Optimization of Freeway Network Operations (August 2021)
On Simulation and Optimization of Freeway Network Operations (October 2021)
On Simulation and Optimization of Freeway Network Operations (February 2022)
On Simulation and Optimization of Freeway Network Operations (May 2022)
On Simulation and Optimization of Freeway Network Operations (August 2022)
Presentations
Wiwatanapataphee, B., Microsimulation of Traffic flow on the Kwinana Freeway with Ramp Metering and Variable Speed Limit, International Conference (UTHM Sciemathic 2020) virtual conference in Malaysia (1-2 December 2020).
Publications
Aljuaydi, Wiwatanapataphee, Wu, et al “Deep learning-based prediction models for freeway traffic flow under non-recurrent events“, 2022 8th International Conference on Control, Decision and Information Technologies (CoDIT), V1, 815-820
Aljuaydi, Wiwatanapataphee, Wu, et al. – “Multivariate machine learning-based prediction models of freeway traffic flow under non-recurrent events“, Alexandria engineering journal V 65, 151-162
Gu, Wiwatanapataphee, Wu, et al. – ” Distributionally robust ramp metering under traffic demand uncertainty“, Transportmetrica
B 10 (1): 652-666
Wang, Wu, et al”A Smoothing Method for Ramp Metering“, IEEE Transactions on Intelligent Transportation Systems 23 (8): 13358-13371, 2022.
Wiwatanapataphee, Wu, Zhang et al. – ” Traffic flow prediction under non-recurrent events using microscopic simulation“, 2022 8th International Conference on Control, Decision and Information Technologies (CoDIT), V1, 506-512
Wiwatanapataphee, Wu, Zhang, et al. “ Long short term traffic prediction under road incidents using deep learning networks”, IEEE 8th International Conference on Control, Decision and Information Technologies Vol 1: 500-505, 2022.
Shortcut summary here : List of various publications 2022
Last Updated: 2024-08-06 14:48:46
Australian Research Council (ARC)
Linkage Project
This project is funded partially by the Australian Government through the Australian Research Council.
—-
September 2018 – August 2021
The primary aim of this project is to develop an innovative approach for improving Road Network Operations under non-recurrent events through big data analysis and computational modelling. Current studies lack sufficient insights into the impact of non-recurrent events, such as accidents and inclement weather, under a seamlessly spatial and temporal context, especially the possible spatial and temporal lag effect due to non-recurrent events. A key focus of the project is to ease the current scientific bottleneck of image and data fusion, analysis and sharing in Road Network Operations. The expected outcome is to optimise traffic control strategies and traffic design, reduce the maintenance costs for road infrastructure and improve quality of life.
Objectives
This project will develop and integrate advanced modelling and computing technologies in spatial and temporal analysis, image and sensing data fusion, and cloud computing to improve the use of data from Intelligent Transport Systems and meteorology monitoring systems. The research will address:
- How to detect non-recurrent events automatically.
- How to assess the impact of non-recurrent events on Road Network Operation variations.
- How to provide real-time updates and share early-warnings to road users.
Industry Outcomes
- For the general public, improvements in Road Network Operation performance monitoring and early warning systems, through efficient modelling, robust analysis and intuitive visualisation, will help road users to avoid congestion and road accidents.
- For the owner/operators, a significantly higher return on investment will be gained through the more reliable digital and modelling methodologies and optimisation of network management.
- For technology providers, adapting image and big data analysis, as well as instant information sharing by cloud computing in Road Network Operations will open a new market in intelligent transport systems, with better information on network needs and capacities.
- Nationally, the improved systems will support a more sustainable transport network that meets the social, economic and environmental needs of today.